Imagine a Smarter World.
An ecosystem that has the maximum amount of diversity is the richest.
Robert Greene
The Writing on the Wall
Both supervised learning and reinforcement learning are insufficient to emulate the kind of learning we observe in animals and humans.
You cannot achieve general intelligence simply by scaling up today’s deep learning techniques.
Pre-training as we know it will end.
The sooner we stop climbing the hill we are on, and start looking for new paradigms, the better.
...current LLMs are not capable of genuine logical reasoning; instead, they attempt to replicate the reasoning steps observed in their training data.
If you look at the improvement from GPT-2 to GPT-3 to 3.5, and then compare that from like 3.5 to 4, you know we really slowed down in terms of the amount of improvement.
Results from scaling up pre-training - the phase of training an AI model that uses a vast amount of unlabeled data to understand language patterns and structures - have plateaued.
AI technology is exceptionally expensive, and to justify those costs, the technology must be able to solve complex problems, which it isn’t designed to do.
Machines’ lack of understanding of causal relations is perhaps the biggest roadblock to giving them human-level intelligence.
I think the progress is going to get harder when I look at '25. The low-hanging fruit is gone. The hill is steeper. You definitely are going to need deeper breakthroughs as we go to the next stage.
Vision
A Smarter World where people and technology work in greater harmony to generate shared prosperity.
Mission
Elevate human potential through intelligent tools that radically improve our mutual understanding.
All intelligence is collective intelligence
In nature, organisms thrive within ecosystems, adapting and maintaining balance. The intelligence of a forest or a reef isn’t isolated—it’s collective, harmonized, and self-organizing.
Nature understands that balance is essential.
People, however, could use a little help.
Inspired by nature’s principles, VERSES is pioneering a new era of AI built on ecosystems of intelligent agents. These agents don’t operate in isolation—they work together, guided by the same principles of harmony and balance that underpin natural systems.
Technology, after all, is an invention of humanity, and humanity itself is a product of nature. By embracing nature’s blueprint of interconnectedness and mutual adaptation, we can create a symbiosis between technology and humanity that is not only efficient but also beautiful, even poetic.
Genius empowers anyone to build agents using a revolutionary algorithm based on natural intelligence: active inference. Agents excel in uncertain and novel situations, anticipating change, and proactively solving problems on their own.
Operating in devices—from IoT to wearables to vehicles and more—these agents continually learn, adapt, and evolve as they interact with the world, becoming experts in their domain. Their focus might be on you and your data or on specific tasks like managing logistics, diagnosing diseases, or driving a barge.
By collaborating, agents can solve problems at any scale, from particle to personal to planetary. They form a distributed network that connects physical and digital domains—a Spatial Web.
The Spatial Web
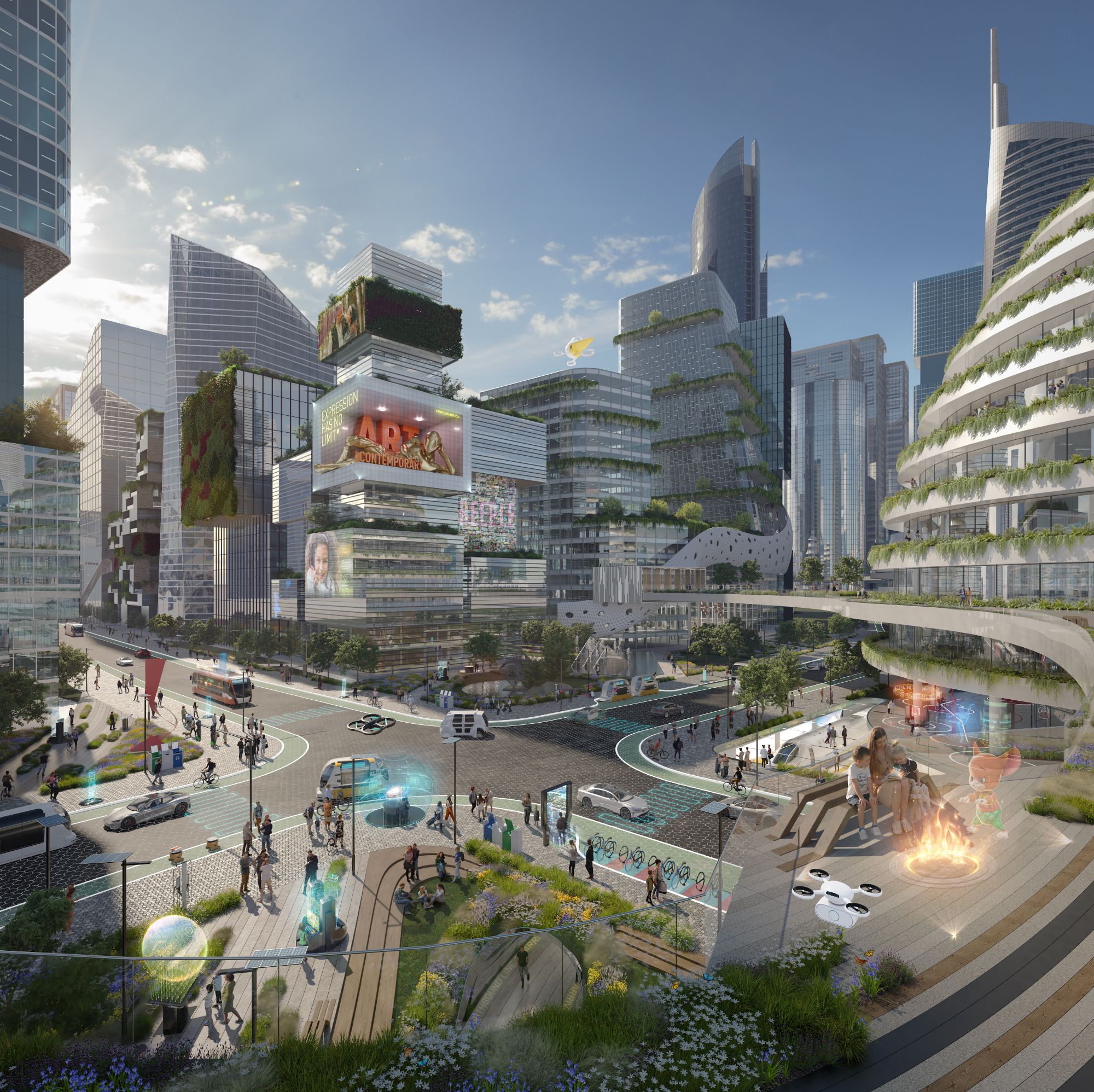
Artificial Intelligence is History
AI models based on the mainstream approach to Deep Learning have improved exponentially in tandem with Moore’s Law and more data.
Until now.
These models are constrained by the quantity and quality of data and improvements are marginal and plateauing. Moreover, once trained, a model is stuck in the past (history).
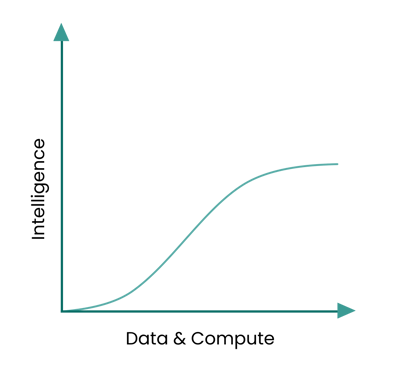
The Future is Fuzzy
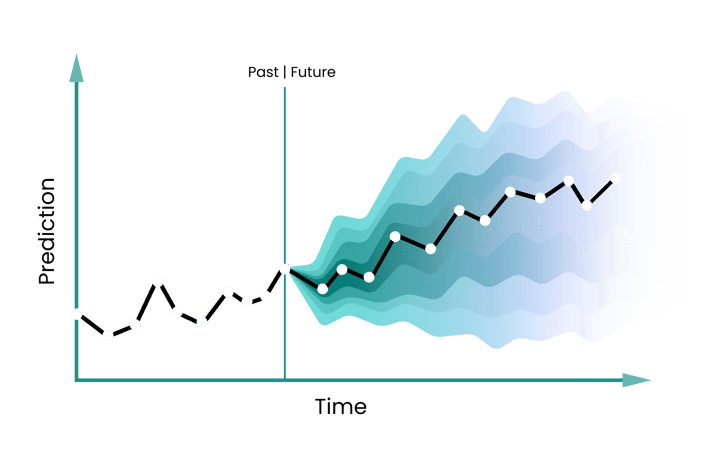
The world is massively complex and dynamic and in order for an intelligent system to make optimal predictions and decisions, its model of the world must factor uncertainty and continuously adapt in light of new information.
Complex Dynamic Systems
Weather prediction, supply-demand forecasting, traffic congestion and routing, insurance modeling, medical diagnosis, and financial markets are examples of incredibly complex systems with intricate interdependencies that make effective and efficient predictions challenging.
Predictions about the world are highly contextual and modeling the fuzziness of knowledge is more effectively represented as probability distributions rather than absolutes.
For example, the model on the right reflects the complexity and uncertainty associated with determining car insurance premiums and payouts.
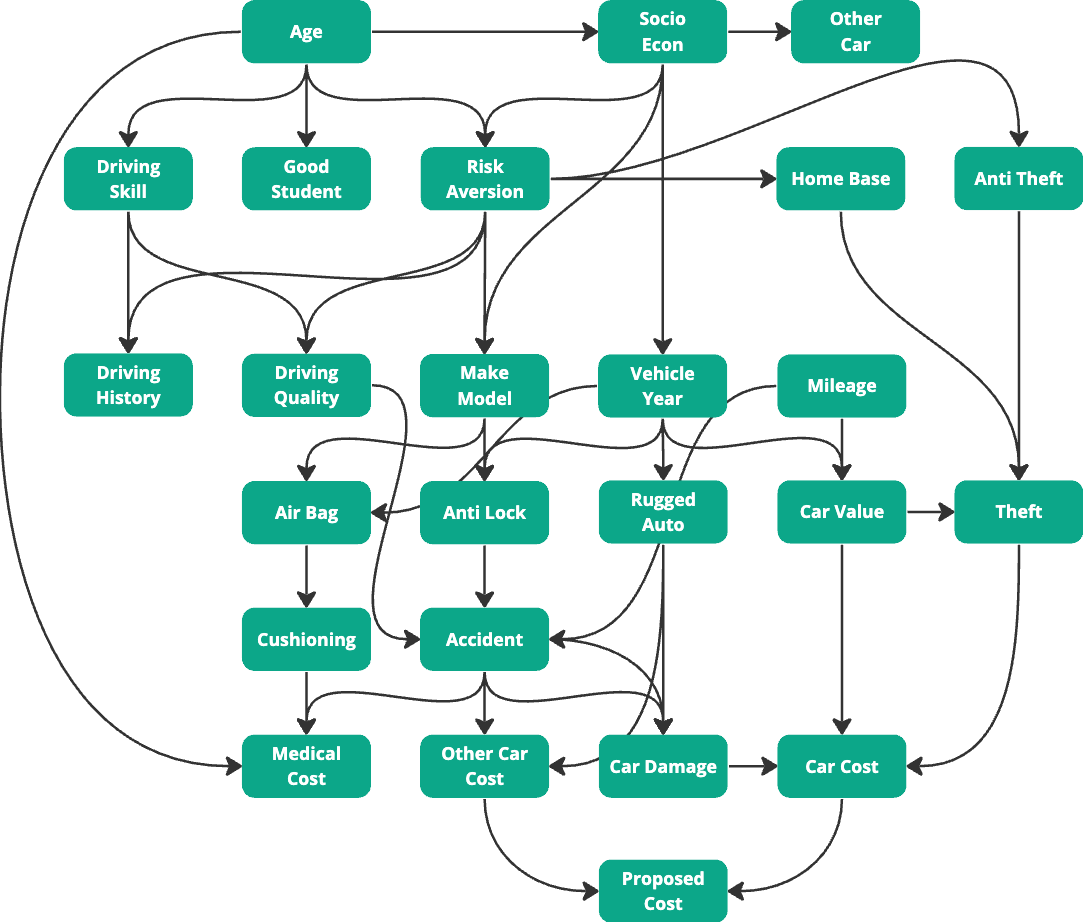
This next-generation web lies at the heart of VERSES’ vision—a foundational shift away from siloed AI systems toward a network of agents functioning as an interconnected whole. Unlike traditional platforms, which silo data and capabilities, this network operates as an open ecosystem, welcoming contributions and encouraging collaboration. It’s a step toward moving beyond fiefdoms (alt. walled gardens) to embrace the true potential of a universal network, just as the open web eclipsed the closed systems of early internet service providers like AOL, Prodigy, CompuServe and MSN in favor of shared innovation.
Achieving this vision requires a new set of open standards and protocols. These tools enable agents to understand the world as we do, work together safely and securely, and contribute to a shared knowledge library called the Universal Domain Graph (UDG).
The UDG is a living, read/write repository that models our world and maps its underlying causal relationships, enabling agents to understand how the world works. Agents can download knowledge models to instantly become experts in any domain. More importantly, they can contribute new insights that improve these models over time.
This continuously updated library unlocks compounding intelligence—a network effect where the entire system learns, adapts, and evolves at a global scale. It’s a resilient, self-organizing system that grows exponentially smarter, functioning like a planetary nervous system, responding to the world’s challenges with unprecedented speed and precision.
And it couldn’t arrive soon enough.
From climate crises to supply chain disruptions, today’s problems are too complex for individuals or isolated systems to solve alone. But this new web of agents offers more than just solutions—it offers reassurance. By embedding intelligence in everything, making it available anywhere, and ensuring it operates in harmony with humanity, we create a future where technology complements rather than overwhelms.
Collective intelligence is the answer: a web of agents working together to address humanity’s most pressing challenges.
An ecosystem of intelligence. A Spatial Web, where intelligence is embedded in everything, available anywhere, for anyone.
Levels of Intelligence
Sentient (S1)
The ability to perceive and respond to the environment in real time. This intelligence is curious and seeks both information and preferences. Such an AI would respond to sensory impressions and be able to plan based on the consequences of an action or belief about the world, which enables it to solve almost any (multiple constraint satisfaction) problem.
Sophisticated (S2)
The ability to learn and adapt to new situations. This intelligence makes plans based on the consequences of an action or beliefs about the world. It moves on from the question of “what will happen if I do this?” to “what will I believe or know if I do this?” This kind of intelligence uses generative models and corresponds to "artificial general intelligence" or AGI.
Sympathetic (S3)
The ability to understand and respond to the emotions and needs of people and other AIs. This type of intelligence has the ability to understand the thoughts and feelings of both humans and other AIs. It can take on the perspective of its users and see things from their point of view. It's often referred to as "perspectival" because it's capable of recognizing and understanding different perspectives, including those of other AIs. It's also described as "sympathetic" since it can comprehend viewpoints other than its own.
Shared (S4)
The ability to collaborate with humans, other agents and physical systems to solve complex problems and achieve goals. It is the kind of collective intelligence that emerges when sympathetic intelligence works together with people and other AI. We believe that this kind of intelligence will come from many intelligent agents (IA) working together, creating — and acting upon — a web of shared knowledge that becomes wisdom.
Sentient (S1)
The ability to perceive and respond to the environment in real time. This intelligence is curious and seeks both information and
preferences. Such an AI would respond to sensory impressions and be able to plan based on the consequences of an action or belief about the world, which enables it to solve almost any (multiple constraint satisfaction) problem.
Sophisticated (S2)
The ability to learn and adapt to new situations. This intelligence makes plans based on the consequences of an action or beliefs about the world. It moves on from the question of “what will happen if I do this?” to “what will I believe or know if I do this?” This kind of intelligence uses generative models and corresponds to "artificial general intelligence" or AGI.
Sympathetic (S3)
The ability to understand and respond to the emotions and needs of people and other AIs. This type of intelligence has the ability to understand the thoughts and feelings of both humans and other AIs. It can take on the perspective of its users and see things from their point of view. It's often referred to as "perspectival" because it's capable of recognizing and understanding different perspectives, including those of other AIs. It's also described as "sympathetic" since it can comprehend viewpoints other than its own.
Shared (S4)
The ability to collaborate with humans, other agents and physical systems to solve complex problems and achieve goals. It is the kind of collective intelligence that emerges when sympathetic intelligence works together with people and other AI. We believe that this kind of intelligence will come from many intelligent agents (IA) working together, creating — and acting upon — a web of shared knowledge that becomes wisdom.
Multi-faceted Intelligence
Our approach is holistic and interconnected, where each form of intelligence builds upon and supports the others. Cognitive capabilities lay the groundwork for effective decision-making, which Physical Intelligence puts into action in the real world. Emotional and Social Intelligence elevate these capabilities by embedding empathy and collaboration, allowing agents to work harmoniously with humans and other agents. Safe Intelligence ensures these abilities are applied ethically and responsibly, while Sustainable Intelligence guarantees that our advancements do not come at the cost of the environment. Together, these six pillars work towards the realization of Shared Intelligence (S4)—an emergent, distributed, and collaborative form of intelligence that benefits humans, society, and the planet.
Our Research Hub provides a deeper exploration into our ongoing projects and the technologies underpinning these six key areas of intelligence. We invite you to explore how we are advancing AI that not only contributes positively to society but also sets a new standard for what AI can and should be.
Select an option below:
- Cognitive Intelligence
- Physical Intelligence
- Emotional Intelligence
- Social Intelligence
- Safe Intelligence
- Sustainable Intelligence
Our work on Cognitive Computing is foundational for all other forms of intelligence. It focuses on developing agents that possess the capacity for causal reasoning, planning, and adaptive learning. Through Bayesian inference, we enable our agents to make decisions that optimize outcomes and reason effectively about their environment. This capability allows agents to anticipate and adapt to future scenarios, contributing to the development of Sophisticated Intelligence (S2) and Sympathetic Intelligence (S3). The ML Foundations Lab plays a key role by optimizing data and memory efficiency, enabling fast and scalable Bayesian learning, while the Formal Methods Lab supports this by providing structured inference rules that promote adaptive decision-making. Cognitive intelligence forms the bedrock that allows agents to think and plan, which is crucial for more advanced interactions.
Building on this cognitive foundation, Physical Intelligence equips agents with the ability to perceive, navigate, and interact within the physical world. Physical intelligence is crucial because it allows agents to embody their cognitive skills in real-world settings. It is not enough for an agent to reason abstractly; it must also apply those concepts through physical actions. This enables embodied action and the practical application of AI in dynamic, real-world environments. The Intelligent Systems Lab is dedicated to enhancing agents' spatial awareness and their capacity to manipulate objects, laying the groundwork for agents that can operate at S2 and S3 levels of complexity. Furthermore, the Ecosystems Lab ensures these physical actions are effectively coordinated across multiple agents, maximizing their collective impact.
Emotional Intelligence builds upon both cognitive and physical abilities by introducing emotional awareness and empathy into agent behavior. This is critical because agents that understand and respond to the emotional and social context of human interaction can create more meaningful and effective relationships with people. By integrating Theory of Mind (ToM) and emotional inference into our models, we aim to align agent actions with the emotions and social contexts of those they interact with, thereby reducing the risk of ethical misalignment and enhancing cooperation. The Intelligent Systems Lab focuses on implementing emotional inference to enhance empathy, ensuring that agent actions are socially aligned. Meanwhile, the Formal Methods Lab supports this effort by developing verification tools that ensure emotionally aware agents behave ethically. Emotional intelligence, therefore, makes AI agents not only more effective in interpersonal interactions but also more aligned with human values.
Social Intelligence is the natural evolution of emotional and physical intelligence, allowing agents to operate in complex, cooperative environments. Social intelligence enables agents to engage in multi-agent, self-organizing behaviors, allowing them to coordinate and cooperate effectively. This capacity to work together—whether with other agents or humans—is essential for creating a cooperative and sustainable ecosystem. The Ecosystems Lab leads our efforts in developing Shared Intelligence (S4), enabling agents to function together in distributed environments. The Intelligent Systems Lab further enhances multi-agent cooperation by ensuring agents can balance individual objectives with collective goals. Social intelligence is what transforms isolated capabilities into collective power, allowing agents to solve problems that are too complex for individuals to tackle alone.
Safe Intelligence underpins every other form of intelligence by ensuring that agents behave in ways that are predictable, explainable, and aligned with human values. Safety is not an afterthought; it is an intrinsic part of our design, supported by Active Inference, which naturally lends itself to transparency. By employing Bayesian reasoning, we enhance the predictability and auditability of decision-making processes. These safeguards prevent misalignment and ensure that AI systems act in the best interest of all stakeholders. The Formal Methods Lab is instrumental in establishing governance standards for agent verification, ensuring compliance with ethical considerations. The Ecosystems lab, focused on governance further embeds ethical safeguards into AI from inception, fostering trustworthiness. Safe intelligence is foundational to ensure that all actions taken by agents are beneficial and aligned with the goals of human collaborators.
Finally, Sustainable Intelligence is an overarching consideration that connects all forms of intelligence to the broader environment in which AI operates. The development of energy-efficient and environmentally sustainable AI is essential to ensure that AI systems can scale without incurring unacceptable ecological costs. By utilizing neuromorphic computing and gradient-free learning, we ensure that our systems are both scalable and sustainable, minimizing their energy footprint while maintaining high adaptability. The ML Foundations Lab works on developing gradient-free biomimetic computations, contributing to efficient and scalable learning models, while the Ecosystems Lab plays a key role in ensuring energy efficiency through distributed architectures and neuromorphic hardware. Sustainable intelligence ensures that as our agents grow smarter, they also grow greener, contributing positively to environmental sustainability.
Autonomous Agent Governance
Autonomous and Intelligent Systems (AIS) present unprecedented opportunities and unprecedented risks that increase as they become more sophisticated. VERSES ecosystem lab specializes in envisioning the governance frameworks and technologies required to mitigate those risks.
Actor Governance
Even today AIS pose technical risks such as the lack of transparency and explainability, fairness biases, misuse, the infringement of privacy and intellectual property rights, and environmental costs. Technical risks can be mitigated reactively by imposing legal safety obligations or sanctions on actors in the AI supply chain. The reactive mitigation of technical risks is called Actor Governance. By conforming to these obligations, VERSES as an actor tries to ensure that its products mitigate such technical risks.
Agent Governance
As AIS become more sophisticated with greater agency and autonomy they will pose new category of risks that include user manipulation and unpredictable harm stemming from the misalignment of the AIS and human goals. Agency risks should be mitigated using governance by design strategies, or “safety valve” technologies that limit harm proactively and this we call Agent Governance. VERSES is actively engaged in developing such technologies for agents [https://arxiv.org/abs/2403.18537].
Network Governance
As AIS evolves into a distributed network of many agents the risks become existential in nature. Agents, collaborating with misaligned goals may make decisions that have large scale deleterious impacts on human populations. Mitigating such risks must be achieved with a Network Governance strategy. By providing mechanisms to monitor and control agent access to data using verification and credentialing systems, Network Governance technologies ensure continuous oversight interactively. This necessitates that all agents on a network, irrespective of its manufacturer, be interoperable. The P2874 Spatial Web Standards allow for such interoperability, credentialling, and verification mechanisms.
RISKS | GOVERNANCE | ENFORCEMENT |
Technical | Actor | Reactive |
Social | Agent | Proactive |
Existential | Network | Interactive |
Ecosystems of Intelligence
The human brain is highly efficient operating on just 20 watts (i.e. enough to power a light bulb). We are generally intelligent biological systems that learn to specialize based on our experiences and we collaborate with each other with individual and shared goals.
This is how we envision the future of technology in the age of intelligence. Not a handful of all-knowing all-powerful systems but rather a vast ecosystem of many efficient, specialized autonomous intelligent agents that thrive in spite of complexity and uncertainty and collaborate on shared goals with shared knowledge while considering the health of the system at all scales.
Read more about our vision in a paper entitled, Designing ecosystems of intelligence from first principles, published in Collective Intelligence.
Here is an executive summary of the paper.
Build a Smarter World with Genius.
Imagine a Smarter World
Model complex dynamic systems and design autonomous intelligent agents.