Smarter by Nature.
Biomimicry is the conscious emulation of life's genius.
Janine Benyus
The Writing on the Wall
Both supervised learning and reinforcement learning are insufficient to emulate the kind of learning we observe in animals and humans.
You cannot achieve general intelligence simply by scaling up today’s deep learning techniques.
Pre-training as we know it will end.
The sooner we stop climbing the hill we are on, and start looking for new paradigms, the better.
...current LLMs are not capable of genuine logical reasoning; instead, they attempt to replicate the reasoning steps observed in their training data.
If you look at the improvement from GPT-2 to GPT-3 to 3.5, and then compare that from like 3.5 to 4, you know we really slowed down in terms of the amount of improvement.
Results from scaling up pre-training - the phase of training an AI model that uses a vast amount of unlabeled data to understand language patterns and structures - have plateaued.
AI technology is exceptionally expensive, and to justify those costs, the technology must be able to solve complex problems, which it isn’t designed to do.
Machines’ lack of understanding of causal relations is perhaps the biggest roadblock to giving them human-level intelligence.
I think the progress is going to get harder when I look at '25. The low-hanging fruit is gone. The hill is steeper. You definitely are going to need deeper breakthroughs as we go to the next stage.
Active Inference
Our research is driven by the vision of creating intelligent systems that are aligned with human values, capable of interacting meaningfully with both humans and the environment, and fostering a more adaptable and sustainable world. We are pioneering a multi-agent framework called Shared Intelligence, which is built upon Active Inference, merging insights from biology, neuromorphic computing, and Bayesian learning principles. The core focus of our research spans six distinct but deeply interlinked areas: Cognitive, Physical, Emotional, Social, Safe, and Sustainable Intelligence.
These themes are not isolated from each other; rather, they are interconnected components of an overarching framework designed to create AI systems that can adapt, learn, interact, and thrive in a dynamic world. Together, they form a cohesive vision for AI that not only solves complex problems but does so while being responsive, ethically grounded, and efficient in resource use.
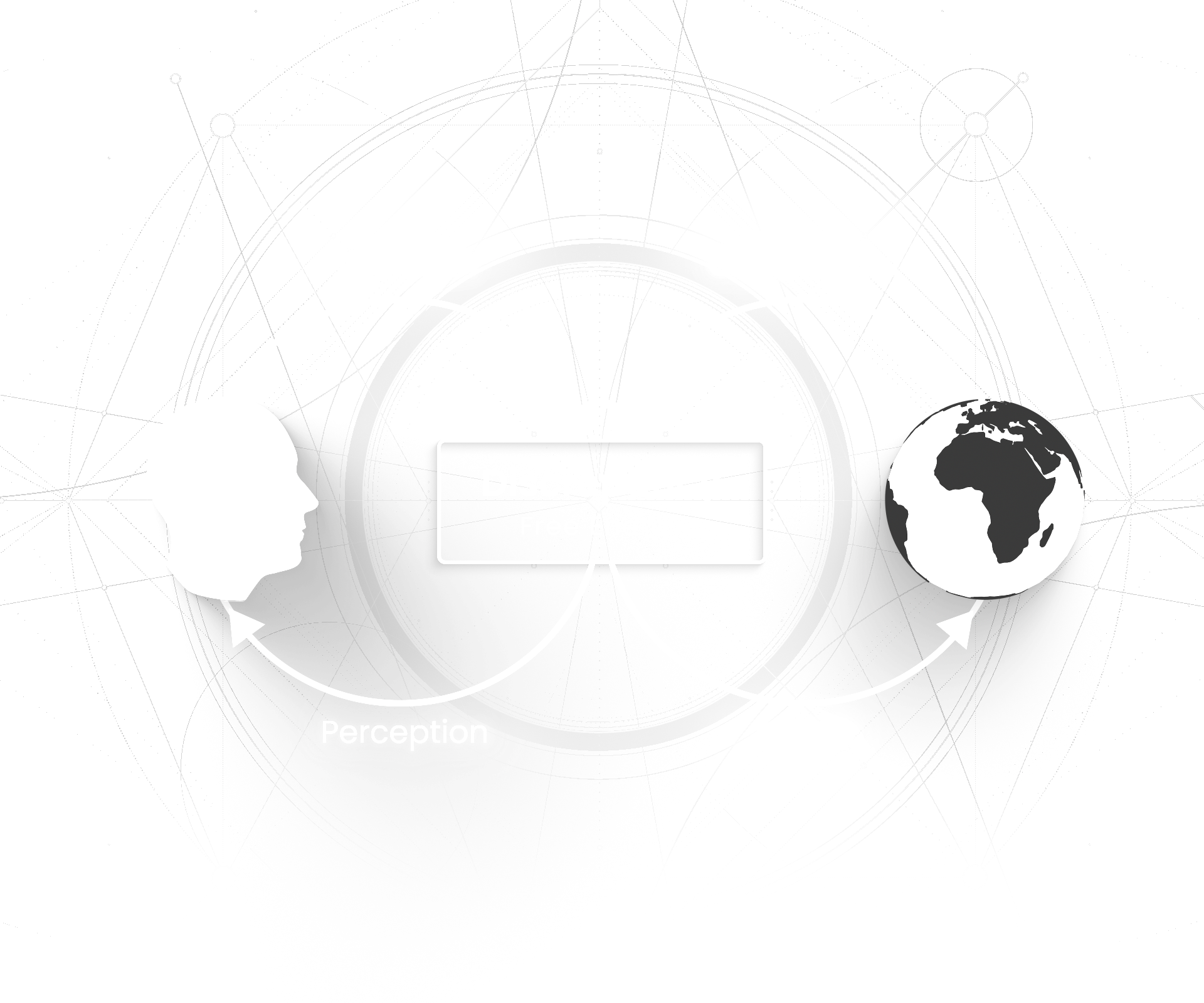
Levels of Intelligence
Sentient (S1)
The ability to perceive and respond to the environment in real time. This intelligence is curious and seeks both information and
preferences. Such an AI would respond to sensory impressions and be able to plan based on the consequences of an action or belief about the world, which enables it to solve almost any (multiple constraint satisfaction) problem.
Sophisticated (S2)
The ability to learn and adapt to new situations. This intelligence makes plans based on the consequences of an action or beliefs about the world. It moves on from the question of “what will happen if I do this?” to “what will I believe or know if I do this?” This kind of intelligence uses generative models and corresponds to "artificial general intelligence" or AGI.
Sympathetic (S3)
The ability to understand and respond to the emotions and needs of people and other AIs. This type of intelligence has the ability to understand the thoughts and feelings of both humans and other AIs. It can take on the perspective of its users and see things from their point of view. It's often referred to as "perspectival" because it's capable of recognizing and understanding different perspectives, including those of other AIs. It's also described as "sympathetic" since it can comprehend viewpoints other than its own.
Shared (S4)
The ability to collaborate with humans, other agents and physical systems to solve complex problems and achieve goals. It is the kind of collective intelligence that emerges when sympathetic intelligence works together with people and other AI. We believe that this kind of intelligence will come from many intelligent agents (IA) working together, creating — and acting upon — a web of shared knowledge that becomes wisdom.
A Bayesian Approach to Intelligence
Our work on Cognitive Computing is foundational for all other forms of intelligence. It focuses on developing agents that possess the capacity for causal reasoning, planning, and adaptive learning. Through Bayesian inference, we enable our agents to make decisions that optimize outcomes and reason effectively about their environment. This capability allows agents to anticipate and adapt to future scenarios, contributing to the development of Sophisticated Intelligence (S2) and Sympathetic Intelligence (S3). The ML Foundations Lab plays a key role by optimizing data and memory efficiency, enabling fast and scalable Bayesian learning, while the Formal Methods Lab supports this by providing structured inference rules that promote adaptive decision-making. Cognitive intelligence forms the bedrock that allows agents to think and plan, which is crucial for more advanced interactions.
Machine learning methods have yielded superhuman performance across a wide spectrum of tasks, invariably driven by the stability and flexibility of a single credit-assignment algorithm: backpropagation of errors (BP). However, despite these successes, this singular commitment to BP has come with widely acknowledged limitations: lack of uncertainty quantification, sample inefficiency, sensitivity to noise, and hardware inflexibility. Naturally intelligent systems like brains suffer none of these problems, operating on fully distributed computational architectures and thriving in noisy and uncertain environments. Thus, there is an increasing need to revisit the foundations of theoretical neurobiology if we are to engineer next-generation AI systems.
There is a growing consensus within the brain sciences that a key to understanding the properties of natural intelligence is to view cognition as a Bayesian inference engine. On this view, natural intelligence constructs probabilistic generative models – or a world model – of its surrounding environment which is used to optimally combine prior knowledge with the incoming stream of data. Active inference is becoming one of the leading theoretical frameworks to describe computation in natural systems. It extends traditional Bayesian accounts of perception, which were first conceived by Helmholtz [] , to encompass action and learning. Unlike typical AI approaches, this theory is underpinned by variational Bayes and implemented as distributed message-passing on probabilistic graphs. Active Inference shares its pedigree with algorithms such as probabilistic signal processing and control-as-inference frameworks and resonates with recent calls in machine learning to adopt more Bayesian principles.
The promise of Bayesian approaches lies in constructing sample-efficient and computationally robust systems that:
- Quantify uncertainty
- Enable policies that actively seek to resolve epistemic uncertainty
- Adapt to changing environments through continual and online learning
- Provide a principled way to reason about data with structured prior knowledge
- Enable fully distributed and local computational architectures through message passing
VERSES is committed to following through on the promise of Active Inference as a foundation for understanding and engineering next-generation AI architectures, but more broadly, to embrace Bayesian (and perhaps post-Bayesian) views on intelligence. In the following blogs, we report our recent progress in this area and describe advancements in our current flagship project: building computationally and sample-efficient architectures for large-scale reinforcement learning challenges.
Multi-faceted Intelligence
Our approach is holistic and interconnected, where each form of intelligence builds upon and supports the others. Cognitive capabilities lay the groundwork for effective decision-making, which Physical Intelligence puts into action in the real world. Emotional and Social Intelligence elevate these capabilities by embedding empathy and collaboration, allowing agents to work harmoniously with humans and other agents. Safe Intelligence ensures these abilities are applied ethically and responsibly, while Sustainable Intelligence guarantees that our advancements do not come at the cost of the environment. Together, these six pillars work towards the realization of Shared Intelligence (S4)—an emergent, distributed, and collaborative form of intelligence that benefits humans, society, and the planet.
Our Research Hub provides a deeper exploration into our ongoing projects and the technologies underpinning these six key areas of intelligence. We invite you to explore how we are advancing AI that not only contributes positively to society but also sets a new standard for what AI can and should be.
Select an option below:
- Cognitive Intelligence
- Physical Intelligence
- Emotional Intelligence
- Social Intelligence
- Safe Intelligence
- Sustainable Intelligence
Our work on Cognitive Computing is foundational for all other forms of intelligence. It focuses on developing agents that possess the capacity for causal reasoning, planning, and adaptive learning. Through Bayesian inference, we enable our agents to make decisions that optimize outcomes and reason effectively about their environment. This capability allows agents to anticipate and adapt to future scenarios, contributing to the development of Sophisticated Intelligence (S2) and Sympathetic Intelligence (S3). The ML Foundations Lab plays a key role by optimizing data and memory efficiency, enabling fast and scalable Bayesian learning, while the Formal Methods Lab supports this by providing structured inference rules that promote adaptive decision-making. Cognitive intelligence forms the bedrock that allows agents to think and plan, which is crucial for more advanced interactions.
Building on this cognitive foundation, Physical Intelligence equips agents with the ability to perceive, navigate, and interact within the physical world. Physical intelligence is crucial because it allows agents to embody their cognitive skills in real-world settings. It is not enough for an agent to reason abstractly; it must also apply those concepts through physical actions. This enables embodied action and the practical application of AI in dynamic, real-world environments. The Intelligent Systems Lab is dedicated to enhancing agents' spatial awareness and their capacity to manipulate objects, laying the groundwork for agents that can operate at S2 and S3 levels of complexity. Furthermore, the Ecosystems Lab ensures these physical actions are effectively coordinated across multiple agents, maximizing their collective impact.
Emotional Intelligence builds upon both cognitive and physical abilities by introducing emotional awareness and empathy into agent behavior. This is critical because agents that understand and respond to the emotional and social context of human interaction can create more meaningful and effective relationships with people. By integrating Theory of Mind (ToM) and emotional inference into our models, we aim to align agent actions with the emotions and social contexts of those they interact with, thereby reducing the risk of ethical misalignment and enhancing cooperation. The Intelligent Systems Lab focuses on implementing emotional inference to enhance empathy, ensuring that agent actions are socially aligned. Meanwhile, the Formal Methods Lab supports this effort by developing verification tools that ensure emotionally aware agents behave ethically. Emotional intelligence, therefore, makes AI agents not only more effective in interpersonal interactions but also more aligned with human values.
Social Intelligence is the natural evolution of emotional and physical intelligence, allowing agents to operate in complex, cooperative environments. Social intelligence enables agents to engage in multi-agent, self-organizing behaviors, allowing them to coordinate and cooperate effectively. This capacity to work together—whether with other agents or humans—is essential for creating a cooperative and sustainable ecosystem. The Ecosystems Lab leads our efforts in developing Shared Intelligence (S4), enabling agents to function together in distributed environments. The Intelligent Systems Lab further enhances multi-agent cooperation by ensuring agents can balance individual objectives with collective goals. Social intelligence is what transforms isolated capabilities into collective power, allowing agents to solve problems that are too complex for individuals to tackle alone.
Safe Intelligence underpins every other form of intelligence by ensuring that agents behave in ways that are predictable, explainable, and aligned with human values. Safety is not an afterthought; it is an intrinsic part of our design, supported by Active Inference, which naturally lends itself to transparency. By employing Bayesian reasoning, we enhance the predictability and auditability of decision-making processes. These safeguards prevent misalignment and ensure that AI systems act in the best interest of all stakeholders. The Formal Methods Lab is instrumental in establishing governance standards for agent verification, ensuring compliance with ethical considerations. The Ecosystems lab, focused on governance further embeds ethical safeguards into AI from inception, fostering trustworthiness. Safe intelligence is foundational to ensure that all actions taken by agents are beneficial and aligned with the goals of human collaborators.
Finally, Sustainable Intelligence is an overarching consideration that connects all forms of intelligence to the broader environment in which AI operates. The development of energy-efficient and environmentally sustainable AI is essential to ensure that AI systems can scale without incurring unacceptable ecological costs. By utilizing neuromorphic computing and gradient-free learning, we ensure that our systems are both scalable and sustainable, minimizing their energy footprint while maintaining high adaptability. The ML Foundations Lab works on developing gradient-free biomimetic computations, contributing to efficient and scalable learning models, while the Ecosystems Lab plays a key role in ensuring energy efficiency through distributed architectures and neuromorphic hardware. Sustainable intelligence ensures that as our agents grow smarter, they also grow greener, contributing positively to environmental sustainability.